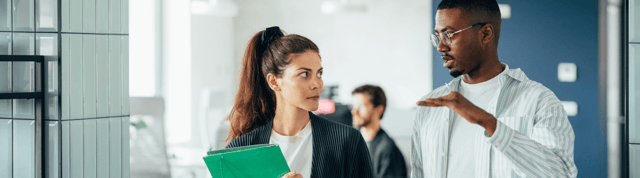
Significance Testing: What Brand Managers Need to Know
If you’re a healthcare brand marketer who uses data, significance testing is a term you’ve probably heard frequently. But what does it actually mean for healthcare marketers, and what’s the best way to use this metric to measure campaigns?
Key takeaways:
- Significance testing tells you whether your data is actionable or not.
- Using a 95% confidence level for significance testing is best practice.
- Sample size matters ¾ larger sample groups produce quality data you can rely on.
Read on for the deeper dive into three best practices related to this important metric.
What you should know
Tests for statistical significance, also known as significance testing, show whether observed differences between results are credible or occur because of sampling error or chance.[i] For marketers, significance testing reveals whether data is actionable or not. When a finding is statistically significant, you can be confident that it’s real and not due to chance.[ii] These findings will tell you whether a particular campaign is effective, allowing you to make informed decisions and yield better business outcomes.[iii]
Knowing more about significance testing can help you make more sound decisions about marketing investments, strategy and resource allocation. A focus on significance testing may not only optimize your marketing efforts but also help you have more productive conversations with your leadership.
Here are three best practices we recommend for you and your analytics partners:
Use the right test in the right circumstance. The great thing about significance testing is that it can be used in many different situations to determine the lift of a campaign. However, it should only be used when comparing at least two groups to each other. Metrics like audience quality, that represent proportions rather than comparisons, are not suited for this kind of testing.
Using test vs. control groups, as in calculating net impact, is a common application of this measurement. The Test group is exposed to a campaign, and the Control group represents people who are not. In other words, we compare people who saw a campaign (Test) to a Control group that acts the way Test would have acted if they had not seen a campaign. A significance test answers the question: Did Test outperform Control? The greater the significance, the less chance that any difference in Test vs. Control is due to random factors.
Pay attention to confidence levels. Using a 95% confidence level for significance testing is best practice. It means that there’s a low chance of error in your results. Unusually low confidence levels ¾ for example, “significant at 57% confidence” ¾ indicate the results are not meaningful and shouldn’t be used exclusively in decision-making.
The lower the confidence level, the greater the chance of seeing a significant difference when it doesn’t exist. That’s called a Type I error. You can also encounter a Type II error, which happens when you miss a potentially significant finding because the confidence interval is too high. Staying with a 95% confidence level will minimize the impact of these issues on your results.[iv]
Consistently use large sample sizes. Generally speaking, the larger your sample size, the more assured you can be that your results are representative of the wider population. This makes larger sample sizes more reliable for decision-making.
Small sample sets are likely to produce misleading results. In this case, the analysis is not valid on a wider scale. It’s been shown that when researchers use small samples, what is identified as a “significant” result is often wrong and may overestimate an effect.[v] For this reason, it’s risky to draw firm conclusions from data taken from smaller groups. This is a challenge in therapeutic areas with limited patient populations such as rare diseases.[vi]
Bonus
These best practices can help you better understand and validate the data provided by your analytics team or vendor. The best analytics experts will partner with you to make sure you’re equipped with quality data and the actionable results you need to drive the best decisions for your brand.
Watch for more upcoming posts on measurement in the weeks ahead. In the meantime, be sure to read “Two Metric ‘Must Haves’ for Healthcare Marketing: Audience Quality and Net Impact.” Or watch our recent webinar, “Our Resident Data Nerd Spills the Tea: Confessions from a Methodologist.”
[i] Institute for Work and Health. Statistical significance. April 2005. https://www.iwh.on.ca/what-researchers-mean-by/statistical-significance.
[ii] Gallo A. A refresher on statistical significance. Harvard Business Review. February 16, 2016. https://hbr.org/2016/02/a-refresher-on-statistical-significance.
[iii] Gell T. Statistical significance in market research [types & examples]. Drive Research. July 10, 2023. https://www.driveresearch.com/market-research-company-blog/statistical-significance-in-market-research-types-examples/.
[iv] Gell T. Statistical Significance in Market Research [Types & Examples]. Drive Research. July 10, 2023. https://www.driveresearch.com/market-research-company-blog/statistical-significance-in-market-research-types-examples/.
[v] Gellman A, Carlin J. Beyond power calculations: Assessing Type S (sign) and Type M (magnitude) errors. Perspect Psychol Sci. 2014;9(6):641–651. http://www.stat.columbia.edu/~gelman/research/published/retropower_final.pdf.
[vi] Mitani AA, Haneuse S. Small data challenges of studying rare diseases. JAMA Network Open. March 23, 2020. https://jamanetwork.com/journals/jamanetworkopen/fullarticle/2763223.